You’re about to tap into a revolutionary way to conduct research, one that streamlines your workflow, enhances efficiency, and boosts accuracy. Leveraging AI in research lets you focus on high-quality, relevant papers, thanks to tools like Consensus and Semantic Scholar that offer semantic searching, vector search, and contextual analysis. With AI summarization, citation mapping, and collaborator network analysis, you’ll gain valuable insights. But how do you make the most of these AI-powered tools? Let’s explore the benefits of AI-assisted research methodologies and see how you can transform your research process.
Key Takeaways
- AI-powered search tools like Consensus, Elicit, and Semantic Scholar leverage NLP and ML to understand search queries and find relevant research papers.
- Semantic search uses techniques like vector search and contextual analysis to encode and analyze query context for accurate results.
- AI filtering and summarization enable focus on high-quality papers and quick comprehension of research through advanced filtering and automated summaries.
- Citation mapping and analysis tools like Research Rabbit and LitMaps visually uncover related papers and identify key authors through citation networks.
- AI-assisted research methodologies offer efficient literature exploration and data analysis, enhancing systematic reviews and research productivity.
Understanding Semantic Searching With AI
Semantic searching with AI is a transformative approach to information retrieval that’s increasingly pivotal in research and various other fields.
By leveraging natural language processing (NLP) and machine learning (ML), semantic search aims to understand the intent and contextual meaning behind a search query, providing more accurate and relevant results.
The key components of semantic search include NLP, which enables computers to understand and process human language, and ML, which trains algorithms to learn from data and improve over time.
This blend allows for semantic understanding, enabling computers to comprehend the underlying meaning of a search query beyond exact keyword matching.
The process involves vector search, which uses text embeddings to encode searchable information into vectors, and contextual analysis, which analyzes the query context to find relevant documents.
This technology is widely used in AI applications such as web search engines, content management systems, and e-commerce platforms to enhance search capabilities.
A critical aspect in implementing semantic search is Data Quality, as high-quality, well-structured data is essential for effective semantic understanding.
Embeddings play a crucial role in semantic search by mapping tokens into a multi-dimensional space where similar tokens are near each other, enabling more precise and relevant search results.
Semantic search algorithms continually learn from user interactions, incorporating personalized search results that adapt to the user’s search history and preferences, further enhancing the search experience.
Citation Mapping With AI Tools
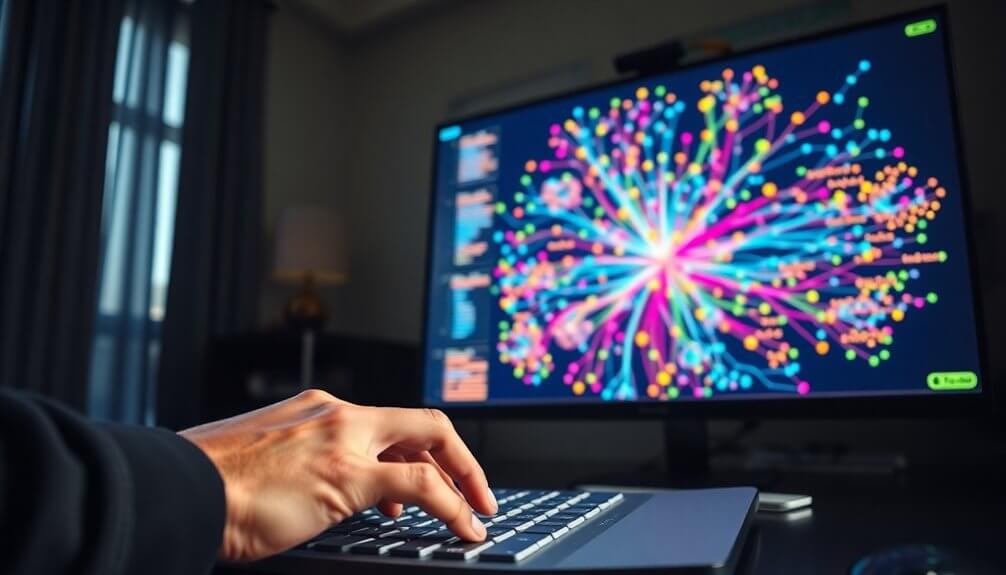
Citation mapping with AI tools comes in. This method uses a visual approach to uncover related papers through a network of citations and citation analysis between scholarly articles.
By leveraging AI-powered citation chaining, you can mine references and citations starting from a single “seed” paper or set of papers, making it easier to identify key publications and authors, and understand the trends in a research field.
Citation mapping tools like Semantic Scholar, LitMaps, Connected Papers, and ResearchRabbit help you navigate citation networks and research landscapes.
These tools create visual maps of related works, prior works, and derivative works, allowing you to efficiently explore relevant literature.
With features like co-citation and bibliographic coupling, you can quickly identify patterns and connections that mightn’t be immediately apparent through traditional keyword searches.
By using citation mapping, you can enhance your literature search, identify trends, and evaluate the reliability of scientific claims. This systematic approach can notably improve your research efficiency and credibility. Additionally, citation mapping tools enable researchers to explore and analyze complex citation networks, identifying influential research and key authors through citation network analysis.
Tools like Scite.ai further enhance this process by analyzing citation context to determine if citations support or dispute the cited claim, offering researchers a more nuanced understanding of scientific reliability through its smart citation index.
Author-Centric Approach Explained
You’re about to explore the author-centric approach, which requires you to ponder the human element in AI design and use. To find key authors, analyze collaborator networks, and identify authorship patterns, you must examine how AI tools can support these tasks while maintaining a focus on human needs and ethical considerations. Human-centered AI emphasizes ethical AI systems that prioritize human values, well-being, and dignity, ensuring AI technologies are developed to augment human capabilities rather than replace them. This approach is grounded in the principle that HCAI systems should prioritize human control, ensuring AI meets human needs while operating transparently, delivering equitable outcomes, and respecting privacy. A data-centric approach, in contrast, involves systematically altering and improving datasets to enhance the accuracy of machine learning applications, highlighting the importance of data quality.
Finding Key Authors
Finding Key Authors (Author-Centric Approach Explained)
Identifying key authors is a crucial step in traversing the vast and dynamic landscape of research literature. By understanding who these key figures are, you can gain insights into the current landscape and future directions of your field. Key authors frequently publish in top-tier journals, have high citation counts, and their work sets the foundation for new research and innovative ideas.
GenAI tools, when used responsibly, can significantly enhance the speed and scale of identifying and evaluating key authors by analyzing vast amounts of data and providing insights that may otherwise have been missed GenAI-assisted research.
Strategies for Identifying Key Authors include leveraging AI tools such as Elicit and ResearchRabbit, which can assist with the automatic identification of authors and related publicationsAI-assisted research. This approach can enhance efficiency and precision in finding influential authors in the field.
Strategies for Identifying Key Authors | Tools and Techniques |
---|---|
Utilize Academic Databases | Google Scholar, Web of Science, Scopus |
Explore Networks of Related Authors and Papers | ResearchRabbit, Connected Papers |
Analyze Citation Counts and h-index | Evaluate an author’s impact through metrics |
Use AI Tools | Elicit, ResearchRabbit, Semantic Search Tools |
To validate your findings, verify an author’s credentials, analyze their track record of publishing in reputable journals, and assess the relevance and quality of their research. By leveraging these strategies and tools, you can effectively identify and evaluate key authors, enhancing your understanding of the research landscape. This thorough approach to finding key authors guarantees that your research is grounded in the latest and most influential works in the field.
Collaborator Network Analysis
Collaborator Network Analysis (Author-Centric Approach Explained)
– Collaborator network analysis is an analytical method that uses co-authorship data to identify key players and networks.
It involves creating co-occurrence matrices from authorship data, which are then analyzed and visualized using social network analysis tools.
Key Insights:
- Identifying central hubs and network cut-points.
- Detecting global collaborations and isolated research communities.
- Informing strategic planning and policy decisions.
- Analysis of neglected tropical disease funding reveals substantial disparities in research investments in Brazil, particularly in diseases such as chikungunya and malaria that have significant prevalence and mortality rates.
- The use of AI literature search tools, such as Semantic Scholar, enhances the efficiency of identifying relevant scholarly articles for network analysis.
Applications of Collaborator Network Analysis:
- Strategic Insights:
- Identifying research partnerships and central institutions.
- Understanding network dynamics and global involvement.
- Capacity Building:
- Recognizing the importance of institutions acting as network cut-points.
- Supporting institutions that connect various parts of the network.
- Network Visualization:
- Creating graphical displays of networks to visualize collaborations.
- Informing decision-making with visual information on network components.
- Tailoring research collaborations based on network analysis.
- Multilevel random-effects models can be used to correct for authorship dependence in results, providing a more accurate picture of the underlying effects.
Authorship Pattern Identification
Technique | Description |
——————————— | ——————————————————————————— |
---|---|
Stylometric Analysis | Examines patterns in written style, punctuation, phrasing, and average word length. |
Linguistically Informed Prompting | Guides LLMs to leverage linguistic features for accurate authorship analysis. |
Similarity Learning | Measures stylistic consistency and divergence between documents and author profiles. |
Classification Models | SVMs, Neural Networks, and Ensemble Models are used for authorship classification. |
In the context of misinformation, verifying authorship becomes critical because unreliable or deceptive information threatens public health, economic well-being, and democracy.
Snowball Method Vs AI Assistance
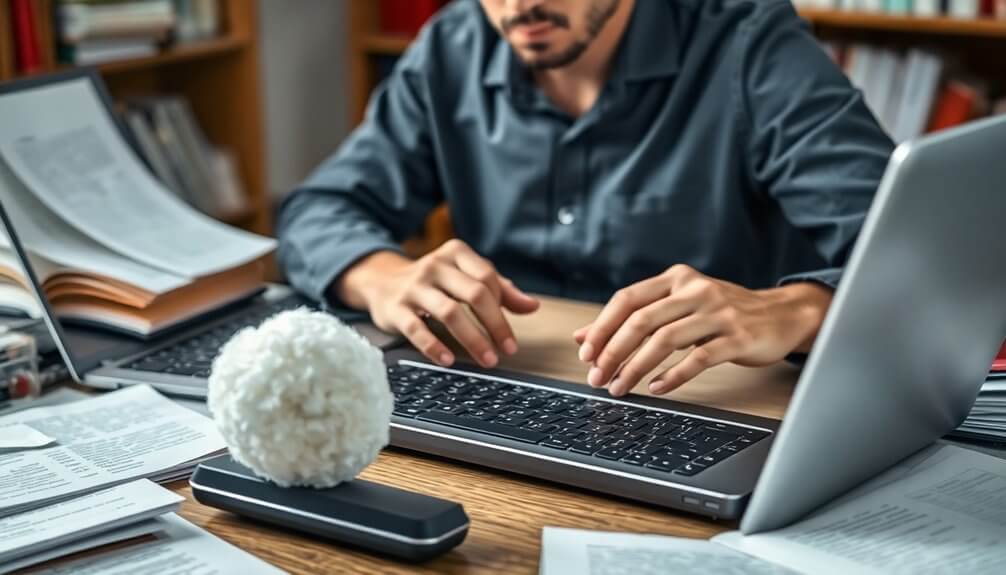
You’re about to set out on comparing the snowball sampling method with AI assistance in research.
While snowball sampling is a non-probability technique that relies on referrals to reach hidden populations, AI tools can automate and enhance research processes such as data collection and literature reviews.
Your critical evaluation will need to ponder factors like time efficiency, thorough literature coverage, and methodological accuracy when deciding between these two approaches.
Snowball sampling is particularly useful for hard-to-reach populations where participants are difficult to locate or contact, making it a valuable tool in certain research contexts. The technique involves ethical considerations, including informed consent to ensure participants are fully aware of the study’s nature and their rights.
AI tools, on the other hand, can provide significant benefits, such as reduced time and effort in conducting literature reviews, thereby increasing research efficiency.
Time Efficiency Comparison
Key points to ponder:
- *Faster Literature Reviews*: AI can identify relevant papers and summarize key findings faster.
- *Automated Data Collection and Analysis*: AI can quickly gather and analyze data, uncovering patterns and trends more efficiently.
- *Improved Writing Processes*: AI tools help with structuring and editing academic papers.
- *Efficient Data Analysis*: AI automates data analysis, arriving at accurate insights faster and at a lower cost.
AI assistance substantially improves the time efficiency of research processes, offering faster methods for literature reviews, data collection, and analysis compared to traditional methods like snowball sampling.
This not only saves time but also reduces the cost of conducting research. AI agents using frontier models have been found to score higher than human experts on average in certain research tasks with short total time budgets, such as 2 hours.
Snowball sampling, a non-probability sampling technique, is often more time-consuming due to its reliance on participant referrals and iterative cycles.
Measuring the efficiency of AI systems is crucial, and it has been observed that algorithmic efficiency gains are multiplicative and can be on a similar scale to hardware improvements over meaningful horizons, highlighting the importance of tracking efficiency trends.
Comprehensive Literature Coverage
While traditional snowball sampling has been effective in identifying hard-to-reach populations and assembling exploratory research, its limitations, such as potential sample bias and difficulty in estimating sampling error, make it less reliable for exhaustive literature coverage.
When you rely solely on referrals from existing participants, there’s a high risk of skewing your sample and missing vital sources. This is where AI assistance comes into play, offering a more thorough and efficient approach to literature exploration.
Additionally, systematic reviews benefit from a well-documented search process, including details about inclusion and exclusion criteria to ensure transparency and reproducibility in selecting relevant studies.
Systematic literature studies have become common in software engineering, emphasizing the need for efficient and reliable methods like snowballing, which involves using article reference lists and citations to identify additional relevant studies.
Methodological Accuracy Impact**
The methodological accuracy of research findings is profoundly impacted by the choice between traditional snowball sampling and AI-assisted research methods.
Traditional snowball sampling involves recruiting participants through referrals, which can lead to biased estimates due to the lack of randomness and overdependence on initial samples.
This method is also subject to sample selection problems due to the homophily principle in social networks, where participants tend to refer individuals similar to themselves.
In contrast, AI assistance can enhance accuracy by analyzing and validating information from multiple sources.
AI tools can detect inconsistencies and errors in data, guaranteeing the originality and credibility of the information.
- Lack of randomness in snowball sampling can lead to measurement error and bias.
- AI assistance can improve data accuracy by cross-referencing multiple sources.
- Traditional snowball sampling is subject to sample selection bias due to the homophily principle.
- AI-driven research methods need transparent and explainable AI to certify trust and reliability.
Overdependence on opaque AI systems in research could lead to unreliable scientific findings, underscoring the importance of maintaining transparency and accountability in AI-assisted research methodologies.
Snowball sampling is particularly useful in accessing hard-to-reach populations, which traditional sampling methods may struggle to reach effectively.
AI algorithms analyze patterns and trends to predict what type of content will resonate most, contributing to higher accuracy in research by identifying the most relevant information content analysis capabilities.
Automated Analysis for Efficiency
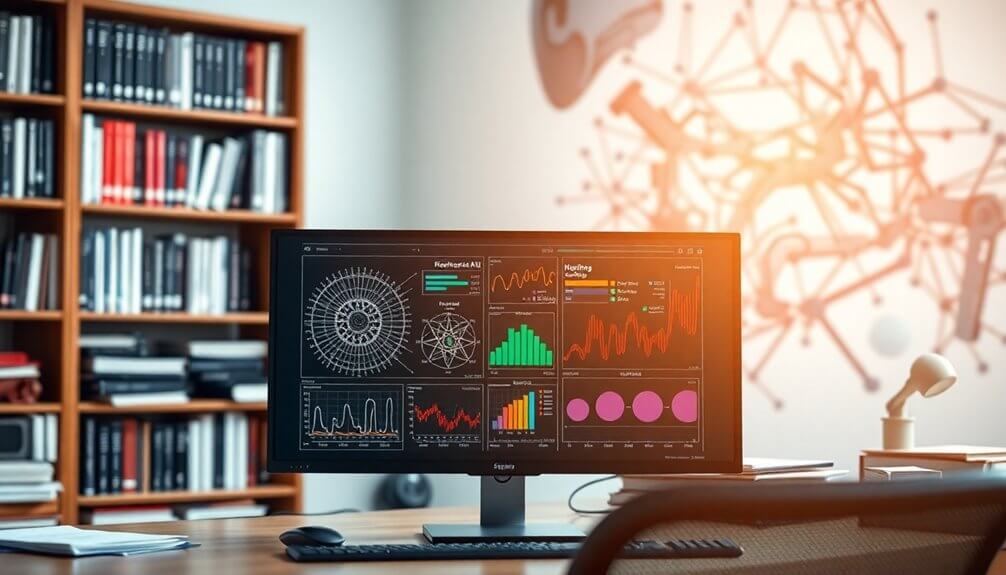
Modern research demands efficient data analysis to stay competitive, and leveraging artificial intelligence (AI) is a crucial step in achieving this efficiency. By incorporating AI into your research workflow, you can automate tedious data preparation tasks, accelerate data analysis, and uncover insights that might have gone unnoticed with manual methods. For example, AI’s ability to handle diverse datasets allows it to effectively process text, images, and high-dimensional data, making it a robust solution for any data preparation task. AI technologies enable smart data analysis by automating tasks such as data extraction and summarization, which further enhances the research process.
| Benefits | AI Capabilities | Research Outcomes
————|—————————————-|——————————–
| Streamlined Data | Automates data cleaning, integration | Increases data quality and reliability
| Efficient Analysis | Analyzes large datasets quickly | Uncovers patterns and correlations rapidly
| Predictive Modeling| Generates precise predictive models | Enhances decision-making with accurate forecasts
| Real-Time Insights | Processes data in real-time | Enables faster and more informed decision-making
| Enhanced Productivity| Automates foundational data tasks | Boosts researcher productivity and efficiency
AI Tools for Literature Search
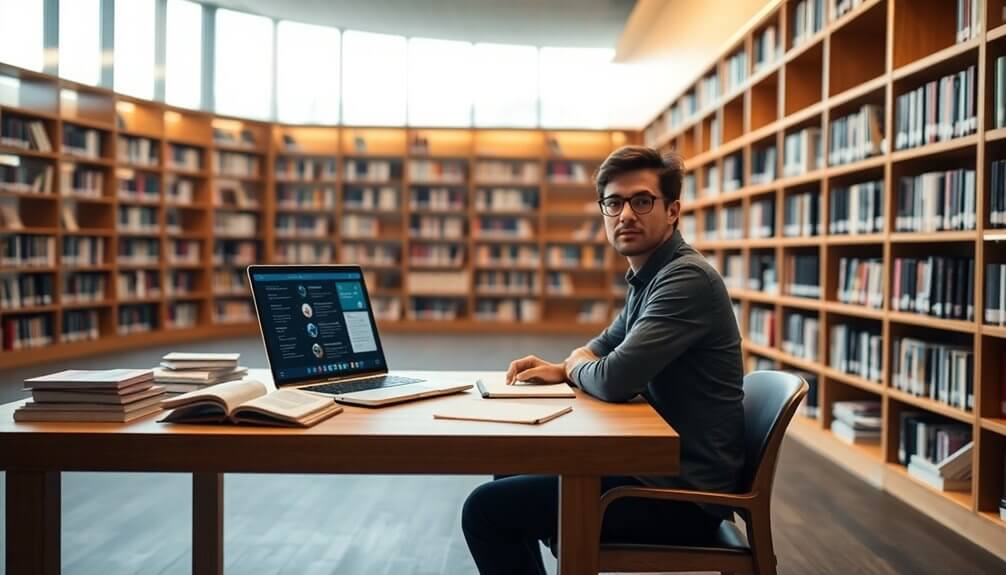
As you plunge into the vast expanse of academic literature, leveraging AI-powered tools for literature search can substantially streamline your research workflow.
With AI literacy, you can navigate and utilize tools like Consensus, Semantic Scholar, and Research Rabbit to optimize your research process.
These AI tools tackle volume overload by efficiently sifting through vast information sources and identifying relevant studies quickly.
For example, Consensus offers advanced filtering by study design, methodology, and more, ensuring you focus on high-quality, relevant papers.
Some key features that enhance literature search include:
- AI Summarization: Automated summaries of research papers for faster comprehension.
- Advanced Filtering: Filter by study design, methodology, and more to refine your search.
- Quality Indicators: Intuitive labels for citations, journal quality, and study type to assess paper quality.
- Interactive Visualizations: Visual tools to explore research connections and networks.
AI in Research Processing
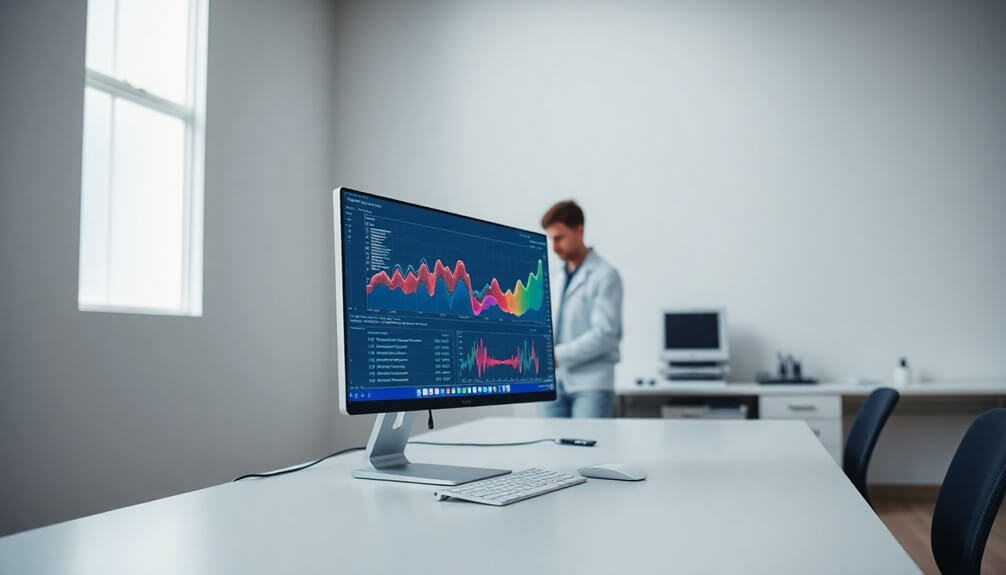
Because leveraging AI in research processing can substantially enhance data analysis and interpretation, incorporating these tools into your workflow is essential. AI-driven data analysis offers numerous benefits, including automated pattern recognition, efficient data processing, advanced statistical analysis, real-time data analysis, and data visualization. These capabilities help you uncover insights that might be missed by human analysts and gain a deeper understanding of complex data.
Key AI Capabilities in Research Processing
AI Capability | Description.related Outcome |
---|---|
Automated Pattern Recognition | Identifies complex patterns in large datasets, enhancing data analysis efficiency. |
Efficient Data Processing | Processes data markedly faster than manual methods, boosting research productivity. |
Real-Time Data Analysis | Enables continuous monitoring and instant analysis of data, providing timely insights. |
Text Summarization | Generates concise summaries of research papers and academic texts, facilitating understanding. |
| Data Visualization | Creates interactive and intuitive visualizations to facilitate comprehension of complex data.
Time Savings and Collaboration
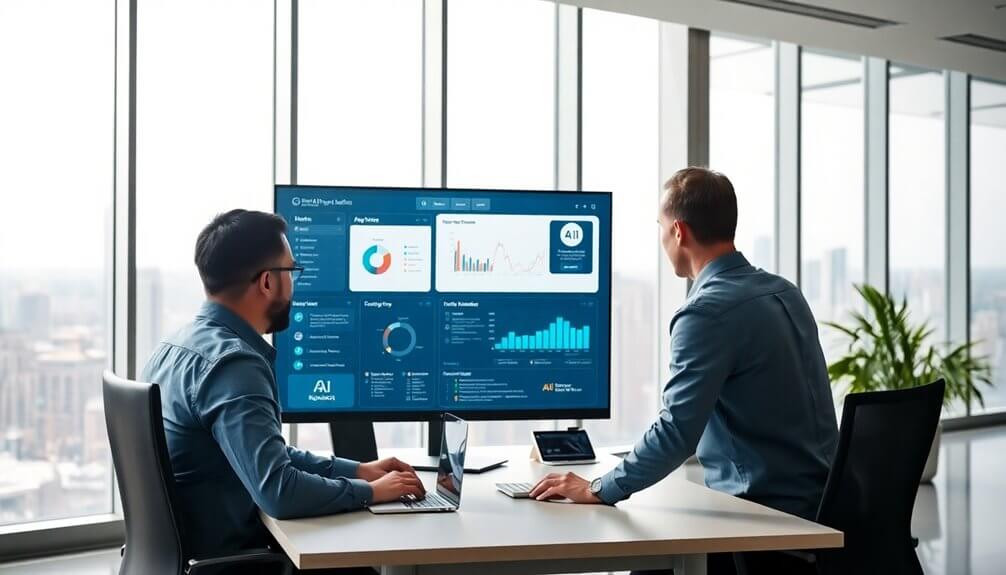
Integrating AI into research processes not only enhances data analysis and interpretation but also substantially impacts time management and collaborative efforts.
By leveraging AI tools, you can save a considerable amount of time that was previously spent on repetitive and labor-intensive tasks. For instance, employees in the energy, utilities, and clean technology sectors report saving an average of 75 minutes daily, while those in the technology and manufacturing sectors save 66 and 62 minutes, respectively.
When you integrate AI into your workflow, you’ll find that you can dedicate more time to high-value tasks like:
- *Quality assurance*: Focusing on checking the accuracy and quality of your work
- *Creative tasks*: Engaging in more creative endeavors that contribute to innovation and problem-solving
- *Strategic thinking*: Using saved time to think strategically about your projects
- *Better work-life balance*: Achieving a healthier balance between work and personal life due to increased efficiency
Leveraging Natural Language Processing
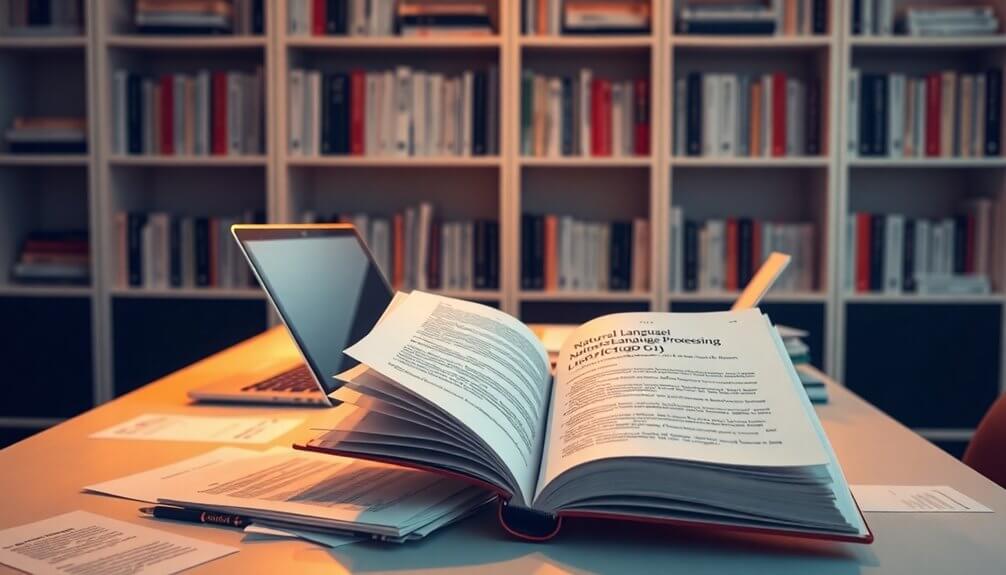
How can you tap the full potential of your research processes by leveraging Natural Language Processing (NLP)?
NLP offers a wealth of opportunities to streamline your research by analyzing vast amounts of data, including unstructured text from social media platforms.
With tools like spaCy, NLTK, and Gensim, you can process text from various sources such as Twitter, Facebook, and YouTube, identifying patterns and sentiments that inform your research.
One critical application of NLP is in social media analysis, where it helps you understand customer opinions and sentiments, identify national security threats, and uncover insights from large data volumes.
For instance, sentiment analysis can categorize user feedback as positive, negative, or neutral, enabling you to make data-driven decisions.
Best Practices for AI-Assisted Research
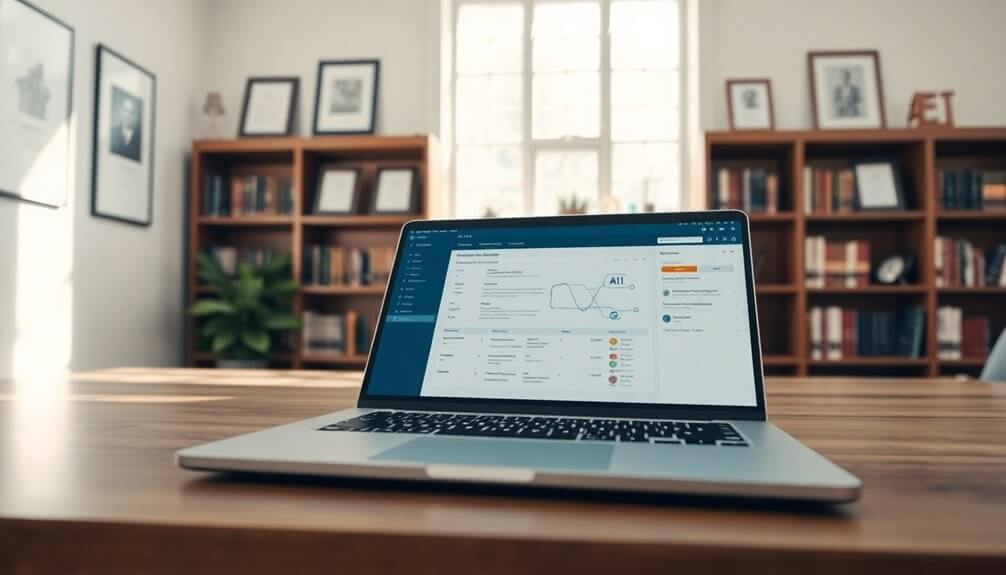
When using AI for research, it’s vital to choose tools that fit your research task and meet ethical standards.
You should evaluate AI accuracy by understanding its capabilities and limitations, and verify tools are transparent about their methodologies and data sources.
As you integrate AI into your workflow, prioritize human oversight and expertise to balance AI efficiency with critical thinking and academic integrity.
Choosing AI Tools
Selecting the right AI tools for research is crucial to leveraging their potential effectively.
When choosing AI tools, you need to ponder several key factors to guarantee they align with your research needs and objectives.
First, assess the compatibility of the AI tool with your current research software and databases.
This integration will streamline your research process and reduce implementation complexity.
Next, evaluate the user interface for ease of use and ponder customization options to tailor the tool to specific research methodologies and requirements.
- Tool Integration: Guarantee the AI tool integrates with current research software and databases.
- User Expertise: Ponder the level of technical expertise of users to guarantee tool usability.
- AI Model Quality: Assess the quality and capabilities of the AI models, pondering model size and training data diversity.
- Scalability: Choose a tool that can scale with research needs, accommodating increasing data volumes without significant additional investment.
Evaluating AI Accuracy
Evaluating AI accuracy is a pivotal step in verifying the reliability and validity of AI-assisted research.
When you use AI tools, it’s paramount to assess the data reliability by evaluating the source and accuracy of the data used to train the AI model. You need to guarantee the data is representative, unbiased, and relevant to your research problem.
Start by validating the data against real-world examples or human judgments.
Understand the limitations of the data and its potential impact on AI outputs. Check if the data is up-to-date and reflects current information.
Next, examine the algorithms used in the AI model for transparency and understandability.
Assess the level of human-reinforced learning in the AI model and evaluate the AI outputs for bias and potential discrimination.
Understand the complexity of the algorithms and their decision-making processes, as well as updates and changes to the algorithms over time.
Integrating AI Safely**
As you integrate AI into your research, adopting exhaustive frameworks such as the Asilomar principles is essential for guaranteeing safety and transparency.
Implementing AI safety frameworks and secure development practices is pivotal to prevent unintended consequences and security breaches.
To integrate AI safely, consider these key strategies:
- Adopt Extensive Frameworks: Implement frameworks that emphasize transparency, accountability, and thorough risk assessment to guarantee AI systems are safe and reliable.
- Conduct Regular Audits: Evaluate AI systems for adherence to established safety standards and regulatory requirements to identify and address potential vulnerabilities.
- Develop Incident Response Plans: Outline clear procedures for identifying, reporting, and mitigating any incidents or breaches to minimize their impact.
- Implement Secure AI Frameworks: Integrate security practices throughout AI system development to protect against unauthorized access and cyberattacks.
Frequently Asked Questions
How Do I Validate the Accuracy of Ai-Generated Summaries?
Start by questioning every AI-generated summary. Cross-reference with original sources, employ fact-checking tools, and engage in critical thinking to identify biases. Then, conduct algorithm auditing and incorporate human evaluation to validate accuracy and certify dependability.
Can AI Tools Handle Non-English Literature Reviews?
You can use AI tools to handle non-English literature reviews, but be aware of language barriers and potential limitations in capturing global perspectives. Tools like DeepL and ChatGPT offer varying degrees of translation accuracy and multilingual capabilities.
How Do I Cite Ai-Generated Content in My Research?
Citing AI-generated content in research is vital for AI accountability and research transparency. Did you know 80% of researchers now use AI tools? To cite AI-generated content: in APA style, treat it as algorithm output with the company as author (e.g., OpenAI, 2023); in Chicago style, use a note or parenthetical citation without a bibliography entry; and in MLA style, describe the generated content, AI tool, version, company, and date, without treating the AI as an author.
Are AI Literature Review Tools Compatible With All Citation Styles?
You’ll find AI literature review tools are generally compatible with major citation styles like APA, MLA, and Chicago, but consider specific tool comparisons and style limitations, especially Chicago’s evolving stance on AI-generated content.
Can AI Tools Identify Biases in Existing Research Literature?**
Can AI tools truly uncover biases lurking in research findings? Yes, AI tools can identify biases in existing research literature, enhancing research transparency and mitigating human oversight through advanced data analysis and machine learning techniques.
Conclusion
Imagine traversing a sea of research papers with ease, thanks to AI-powered tools that streamline your workflow. With semantic searching and citation mapping, you plunge into the depth of academic knowledge, uncovering relevant papers and insights like treasures hidden beneath the waves. By leveraging AI in research, you save time, enhance accuracy, and open doors to new discoveries, transforming the way you work and collaborate. AI literacy becomes your compass, guiding you toward high-quality research.